Notice
Recent Posts
Recent Comments
Link
일 | 월 | 화 | 수 | 목 | 금 | 토 |
---|---|---|---|---|---|---|
1 | 2 | |||||
3 | 4 | 5 | 6 | 7 | 8 | 9 |
10 | 11 | 12 | 13 | 14 | 15 | 16 |
17 | 18 | 19 | 20 | 21 | 22 | 23 |
24 | 25 | 26 | 27 | 28 | 29 | 30 |
Tags
- deep daiv. 2주차 팀 활동 과제
- deep daiv. week3 팀활동과제
- deep daiv. project_paper
- deep daiv. week4 팀활동과제
- deep daiv. WIL
Archives
- Today
- Total
OK ROCK
[NLP] Information Retrieval(3): Vector-based Retrieval 본문
< Week 3 Contents > 中
1. Keyword-based Retrieval
2. Evaluation Metrics
3. Vector-based Retrieval ☜
~ week3의 마지막 내용입니다. 코드 중심
Theory
vector간의 코사인 유사도를 계산하여 가까운 것끼리는 비슷한 의미를 나타나게끔 projection하는 방법을 이용합니다.
Code
1. Embedding based Methods
(model과 tokenzier는 모두 transformer의 Auto 버전으로 불러들였습니다. 생략)
(1) 입력한 텍스트를 배치로 갖는 set에서 model input&output 확인
1
2
3
4
5
6
7
8
9
10
11
12
13
14
15
16
17
18
|
text = "그러나 사실 피고인은 D은행에서 대출을 받아서 돈을 갚을 생각이 없었고"
model_inputs = tokenizer(text, max_length=1024,
padding=True,
truncation=True,
return_tensors='pt')
out = model.generate(
model_inputs["input_ids"].to(device),
max_new_tokens=150,
pad_token_id=tokenizer.pad_token_id,
use_cache=True,
repetition_penalty=1.2,
top_k=5,
top_p=0.9,
temperature=1,
num_beams=2,
)
|
cs |
출력 결과)
(2) 전체데이터를 감염병 / 근로 set으로 split & Batch처리하고, 위와 같은 과정 수행
1
2
3
4
5
6
7
8
9
10
11
12
13
14
15
16
17
|
batch_size = 4
input_texts_disease = [x["facts"] for x in data_disease[:batch_size]]
input_texts_labor = [x["facts"] for x in data_labor[:batch_size]]
features = tokenizer(
input_texts_disease, #감염병관련 법안 batch처리한 data
max_length=1024,
padding=True,
truncation=True,
return_tensors="pt",
)
out = model(
input_ids=features["input_ids"].to(device),
attention_mask=features["attention_mask"].to(device),
output_hidden_states=True
)
|
cs |
(3) Average Pooling
1
2
3
4
5
6
7
8
9
10
11
12
13
14
15
16
17
18
19
20
|
embs_disease = last_hidden_state.mean(dim=1)
#감염병 데이터 pooling 완료!
embs_disease.shape # torch.Size([4, 768]) 출력됨
# 근로관련데이터(labor)에서도 pooling까지 같은 과정 반복합니다.
feature = tokenizer(
input_texts_labor,
max_length=1024,
padding=True,
truncation=True,
return_tensors="pt",
)
out = model(
input_ids=features["input_ids"].to(device),
attention_mask=features["attention_mask"].to(device),
output_hidden_states=True
)
last_hidden_state = out["hidden_states"][-1]
embs_labor = last_hidden_state.mean(dim=1)
#근로, 노동관련 데이터 pooling 완료! |
cs |
(4) batch_size 증가시키고 전체과정 수행(사실상 이것만 돌려도 됨)
1
2
3
4
5
6
7
8
9
10
11
12
13
14
15
16
17
18
19
20
21
22
23
24
25
26
27
28
29
30
31
32
33
34
35
36
37
38
39
|
with torch.no_grad():
batch_size = 24 # 증가되었죠?
input_texts_disease = [x["facts"] for x in data_disease[:batch_size]]
input_texts_labor = [x["facts"] for x in data_labor[:batch_size]]
# st = i * 6
# ed = st + 4
features = tokenizer(
input_texts_disease,
max_length=1024,
padding=True,
truncation=True,
return_tensors="pt",
)
out = model(
input_ids=features["input_ids"].to(device),
attention_mask=features["attention_mask"].to(device),
output_hidden_states=True,
)
last_hidden_state = out["hidden_states"][-1]
embs_disease = last_hidden_state.mean(dim=1) #완료!
features = tokenizer(
input_texts_labor,
max_length=1024,
padding=True,
truncation=True,
return_tensors="pt",
)
out = model(
input_ids=features["input_ids"].to(device),
attention_mask=features["attention_mask"].to(device),
output_hidden_states=True,
)
last_hidden_state = out["hidden_states"][-1]
embs_labor = last_hidden_state.mean(dim=1) #완료!
|
cs |
출력 결과)
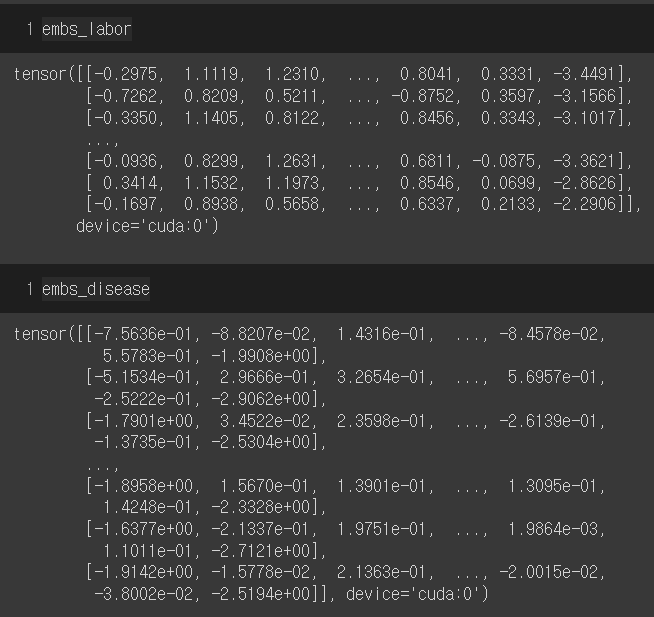
(5) 각각의 vector를 concat시켜서 하나로 표현 (t-SNE 쓰기 위해)
1
2
3
|
embs = torch.cat([embs_disease, embs_labor], dim=0)
embs.shape #전체 embedding vector를 합쳐줍니다.
# 차원 출력 결과, torch.Size([48, 768]) 왜냐면 batch size 24
|
cs |
(6) t-SNE사용하여 embedding space 시각화
1
2
3
4
5
6
7
8
9
10
11
12
13
14
15
16
17
|
# t-sne method
from sklearn import manifold
tsne = manifold.TSNE(n_components=2, init="pca", random_state=0)
X_tsne = tsne.fit_transform(embs.detach().cpu().numpy())
import numpy as np
import matplotlib.pylab as plt
a, b = X_tsne.T
a = (a - np.min(a)) / ( np.max(a) - np.min(a))
b = (b - np.min(b)) / ( np.max(b) - np.min(b))
plt.figure()
labels = ['d']*batch_size + ['l']*batch_size
colors = ['r']*batch_size + ['k']*batch_size
for a1, b1, label, color in zip(a, b, labels, colors):
plt.text(a1, b1, label, color=color)
|
cs |
2. Using FAISS 라이브러리( Facebook AI Similarity Search )
References
- ※
개인 공부용으로, 코드 공유 금지
'Study > NLP' 카테고리의 다른 글
[NLP] Text Generation(1) : Decoding (0) | 2023.10.13 |
---|---|
[NLP] Information Retrieval(2): Keyword-based Retrieval (0) | 2023.09.30 |
[NLP] Information Retrieval(1): Evaluation Metrics (0) | 2023.09.27 |
[NLP] Byte-Pair Encoding tokenization (5) | 2023.09.17 |